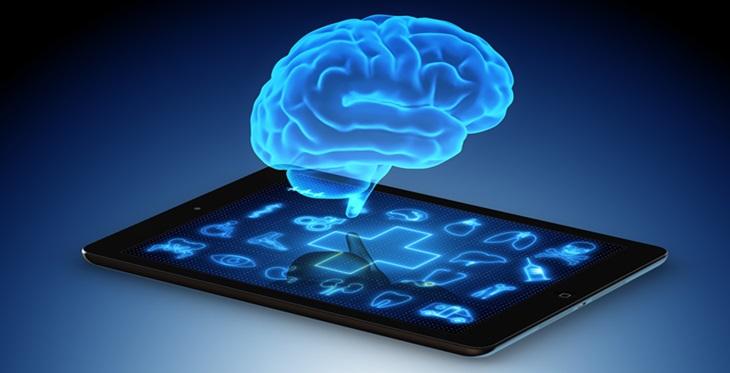
The current and future potential impact of Artificial Intelligence (AI) on healthcare, particularly in relation to telemedicine, is huge. Presented here are just a few of the more exciting technologies and their potential for use and impact in telehealth applications.
With ambient clinical listening technologies (AI-based scribes), advanced, voice-enabled AI tools automatically document patient encounters and free-flowing conversation between physicians, patients, and families. An advantage of using AI scribes in a telemedicine setting is that providers can focus more continuously on their consultation with the patient, eliminating the need to divert their attention away from the patient for note taking. Using AI scribes can save providers significant amounts of time as the burden to create accurate notes or encounter summaries in the electronic health record is reduced as it can be done automatically with minimal editing. Some AI scribe products also include predictive tools that can analyze the conversations, provide feedback, and assist with order placement, future appointment scheduling, or prescriptions, which can help reduce provider burnout, save time, and help ensure that all topics discussed are properly coordinated.
Embodied AI technology, where AI is integrated into robots with various sensors that allow them to interact with their surroundings and perform tasks, can be used in many clinical settings, such as visiting patients and allowing providers to connect virtually to the patient through the robot - acting as a surrogate for in-person providers during rounds, for example. The sensors can import data from the surroundings that the robot can analyze and learn from to interactively and iteratively reach a given goal. They develop abstract representations and understanding of spatial and temporal dimensions in the world to solve complex tasks (e.g., preventing or helping a patient from getting out of bed).
There is a very significant role for AI in the healthcare sector, particularly in electronic medical records. AI tools can conduct predictive analyses, data visualization, collect and sort patient data, create visualizations, and predict potential health issues. Natural language processing (NLP) tools can be used to simplify complex medical reports into understandable language for both patients and healthcare providers. AI can help predict patient outcomes, mobilize data between different systems, match patients (e.g., multiple records from different providers and services to reduce wrong patient errors), and increase compliance by sending automatic reminders to patients and providers to improve rates of follow-up visits and reduce no-shows. AI can also enhance health information exchange, improving billing accuracy, and triaging patients for telemedicine visits.
The integration of AI into wearables (e.g., shirts, socks, rings, watches) for monitoring various health metrics is already improving patient care. AI can analyze large amounts of data collected from patients and their devices to establish their baselines (e.g., heart rate, A1C levels, respiration), enabling the detection of deviations and potential health issues. Some can even notify providers of potential adverse events. There are wearable technologies (socks with embedded sensors) for tracking patients, such as those with dementia or Alzheimer's, that can alert caregivers if they wander away. These technologies are already being widely used by the general public in many wellness applications and demand for integration into the health record and healthcare will only grow.
There is great utility in using voice recognition, sensors, and other AI-based tools for analyzing patients’ voices and facial expressions, helping to detect, for example, mental health issues. Biomarkers, like subtle changes in one’s tone of voice, volume, and other parameters that might be less noticeable during a telemedicine visit can be detected by the AI that can then alert the provider to possible depression, anxiety, or lack of emotion. Facial recognition tools have similar capabilities in detecting emotional states that could be indicators of underlying mental or behavioral health issues that the provider may miss. There are even AI tools that can track and analyze a person’s gait. This could be very useful for telerehabilitation visits where the therapist can watch the person on the computer monitor but may not see enough detail to fully recognize changes or problems in gait that might have been more obvious if the visit was in person.
There are, however, challenges associated with the implementation of AI systems in healthcare and telemedicine. Many health systems lack internal AI expertise, do not fully understand associated compliance and regulatory issues, and user trust is often a significant obstacle. AI systems do make mistakes (e.g., hallucinate, are not always accurate) so we need to ensure their accuracy, as well as their fitness for the work that we give them, to avoid potential diagnostic errors. There are also concerns about the return on investment as the business models for how to purchase and integrate these tools into existing workflows and systems are often not existent or not well developed. There are some Current Procedural Terminology (CPT) codes available for AI use, but they do not cover all the potential areas where AI can be used clinically, thus healthcare systems must decide if the investment in purchasing or licensing these tools will result in other cost savings (e.g., fewer admissions, reduced no show rates) that outweigh the lack of reimbursement options. Information security measures and policies for AI systems used in healthcare settings are critically important. AI systems are not immune from vulnerabilities common to computing technologies. Training the workforce in the allowable and appropriate uses of AI is essential to developing and maintaining robust information privacy and security programs.
There are also potential ethical issues – who owns the healthcare data needed to train these tools, should data be shared and with whom, should AI operate independently of humans making their own clinical decisions? It is critically important to train AI systems with diverse populations to avoid biases and ensure accurate results, but how do we get access to data from these populations? There are also concerns about the quality of the data used to train these systems and the relevance of certain performance metrics. Is it enough for a system to provide an answer quickly or should we be more concerned about accuracy and the impact the outputs of these tools have on patient outcomes? These are just a few of the questions that have arisen recently as AI becomes more of a reality in healthcare.
There are additional potential challenges of using artificial intelligence (AI) in healthcare, particularly in telemedicine. The "black box" nature of AI, where the system's decision-making process is opaque, can make it difficult for healthcare professionals to act on the information it provides. They need to be able to trust the information being provided by an AI is valid, accurate, and reliable. Finally, there is a growing concern about de-skilling, where the use of AI tools can lead to a reduction in valuable skills among healthcare providers. We need to address these challenges before AI can be fully integrated into healthcare practice. It is a journey, and we are already well down the AI highway with some clear advantages and benefits being realized.