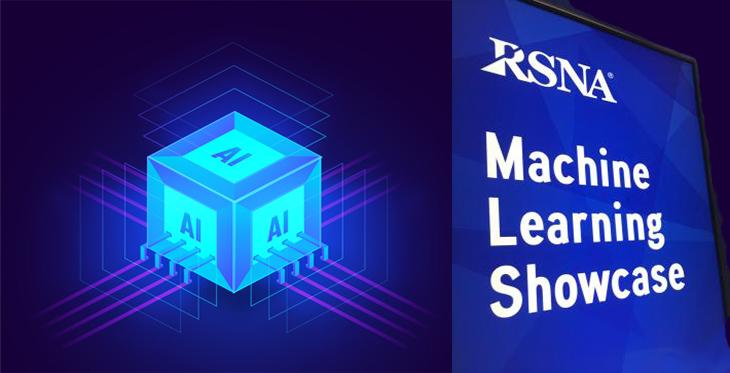
Practically everywhere you turn today, AI, or artificial intelligence, (aka deep learning and machine learning) pops up as the must have, coolest thing since robots and thinking machines were first introduced in popular literature and films. After all, who doesn’t want a car that can sense when it’s safe to change lanes, stop before hitting the deer in the road and even drive itself?
There is little doubt that AI already has and will continue to revolutionize the world and thereby healthcare. For example, the past five years at the Radiological Society of North America (RSNA) annual meeting -- the world’s largest radiology meeting, attracting over 50,000 people to Chicago -- have seen an explosion in the number of vendors promoting AI in their products, and the number of scientific talks and courses on the topic. The 2018 meeting in November was no exception – I swear, every single vendor must have had “AI” advertised somewhere on their booth. Throughout the meeting sessions, AI, deep learning and machine learning topics permeated presentations.
The 2018 Radiological Society of North America (RSNA) meeting in Chicago highlighted AI, deep learning and machine learning in numerous sessions.
Today, teleradioloy and radiology are practically synonymous, so whatever impacts radiology impacts teleradiology. The question is, however, where will it not (or perhaps should not) do what many are afraid it will – take over the roles and responsibilities of radiologists, medical physicists and others involved in the radiology and teleradiology enterprise? You’re thinking – could AI really replace radiologists? There are many who believe the answer is yes (see opinions by Mutaz Musa in The Scientist https://www.the-scientist.com/news-opinion/opinion--rise-of-the-robot-radiologists-64356; and Obermeyer & Emanuel https://www.nejm.org/doi/full/10.1056/NEJMp1606181). Thus, reactions among practicing and future radiologists to AI tend to range from horror to ardent enthusiasm. Is AI really the harbinger of doom for the practicing radiologist, simply the latest gee whiz computer geek fad, or is it possible that it may actually have a positive role in radiology and healthcare in general? I tend to fall in with those who think it will have a positive impact – but it will not replace the radiologists or other key personnel.
AI can help provide valuable and accurate information with respect to a multitude of essential care variables. For medical physicists it has clear roles in image repeatability and reproducibility; adaptive sequence generation; automated protocolling; assisting with smart positioning to decrease retakes; dose reduction; and assisting with treatment planning. For radiologists, the applications are numerous as well, and these can directly influence the practice of teleradiology and patient care. The most common, or at least visible, AI application is image segmentation and analysis to detect and classify (e.g., benign vs malignant) radiographic findings. There are a multitude of studies being published in a wide variety of journals on how well a given AI algorithm does on a particular clinical image type and lesion target, often with fairly impressive results. Many of the authors conclude their papers by claiming their algorithm outperforms radiologists. AI is really good at the tedious tasks a radiologist often has to do but increasingly does not have the time to do. For example, measuring the size of lesions, especially as they change over time do to treatment (e.g., RECIST measurements) is tedious, time consuming and fatiguing. If an AI algorithm can do this in a consistent and reliable manner and automatically import the final values into the report – let it! Let the radiologist make the complicated decisions regarding what those measurements mean and what the implications are for the next treatment steps.
With these image-based deep learning studies, however, relevant questions must be asked:
a) How subtle are the findings under consideration (e.g., were they missed during clinical interpretation)?
b) How difficult are the “normal” cases (e.g., is there opportunity for the tool to make false positives)?
c) Is there really a need for such a tool in clinical practice (e.g., is the task associated clinically with poor sensitivity and or specificity)?
d) Was the task chosen because a set of images with a given abnormality happened to be readily available (as is often the case with studies using mammographic images)?
e) Where did the radiologist performance data come from (e.g., experienced vs trainee readers, sub-specialty or general radiologists, single radiologist or a panel).
These types of questions should always be considered when deciding whether the results of a deep learning investigation are likely to have an impact on clinical practice.
Other areas where AI is being applied in radiology include the use of natural language processing (NLP) to help convert free text reports to structured formats that might be more readily interpreted by referring clinicians and patients, clinical decision support tools, predictive analytics, clinical trial enrollment, quality control initiatives, workflow analytics and a host of other important and exciting applications.
From my perspective, the next phase in all of this is practical usability. How can we make these tools readily available and in a “friendly” and clinically useful form? For teleradiology, I think there are some immediate applications. For example, small rural clinics often use teleradiology because the on-site radiologist is a general radiologist, thus more complicated cases (e.g., neuroradiology) require sub-specialist interpretation so the images get transferred to a remote sub-specialist radiologist for interpretation. AI’s role in this situation could be to provide the on-site general radiologist with some decision support tools and outcome predictions in a more efficient manner than an off-site radiologist could but who would still provide a read of the case for the final interpretation and recommendations (much like when a resident completes the preliminary read and the radiologist signs off on it).
If these AI tools are to be used clinically, we need to integrate them into the workflow by assessing where, when, and how they will be most effectively used without adding extra burden to a radiologist’s already complex decision-making environment. Future work needs to address two other fundamental issues related to integration and effective and efficient implementation. The first is that the tools (and hence the evaluation methods) need to move beyond binary decisions (disease present/absent, malignant/benign) if they are going to truly aid the radiologist in the complex decisions that need to be made that are often not binary. The second is, perhaps, more complicated. The majority of (if not all) deep learning techniques developed to date are uni-taskers. They address a single type of image/modality and a single disease entity. There are likely situations where this is perfectly appropriate and useful, but in the long run we cannot have a plethora of different independent schemes providing a multitude of “opinions” that the radiologist needs to somehow sift through to make sense of. Future research will need to develop ways to integrate and prioritize these various outputs; they must be presented to the radiologist in a fashion that makes sense, does not contribute to information overload, and actually improves the decision-making process.
Why else won’t AI replace radiologists, medical physicists and other key healthcare team members? What it cannot do (at least not yet) is engage in team consultation to explain the reasons behind a given decision or proposed method of completing a given clinical task, or modify these decisions based on collaborative and interactive input derived from the knowledge and clinical experience of other team members and the uniqueness of each clinical encounter and patient. Deep learning and AI are still a long way from being creative and this has been the case from the very beginning of AI implementations. As Boden pointed out in 1998, the two major bottlenecks to AI creativity are domain expertise and valuation of results (critical judgment of one’s own original ideas).
I think AI still has not been able to master these hurdles and display true creativity. Radiology is all about solving complicated clinical problems, developing new lines of research investigation, and communicating and collaborating with colleagues and patients – all of which involve creativity and ingenuity. Let the computers take over the tedious, monotonous and time-consuming tasks. Humans will have more time to create, discover and lead healthcare to next level.