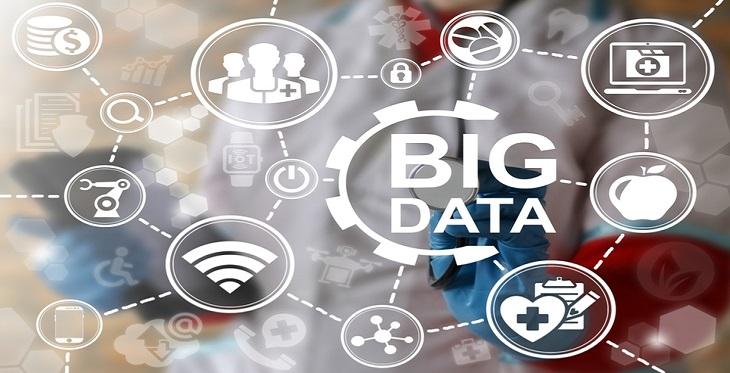
A central feature of Telehealth is that data, potentially in vast amounts, are accumulated about patients by provider organizations. This data has a variety of important uses beyond the diagnosis and treatment of each specific patient, both for individual health organizations and for the public at large (e.g., population health). Without modern data management technology, namely a data warehouse, there is no efficient way to analyze data aggregated from large patient populations, or ultimately use it to support data-driven healthcare decisions.
A data warehouse is a central data repository that stores data from multiple sources across an organization, enabling organizations to extract useful information.
Organizational data is usually spread out across many disparate systems and formats, making it difficult to analyze and extract meaningful information. Data warehouses aggregate data into a structured format that can easily be queried. A data warehouse can be structured as “on premise system” or as a “cloud-based service”. Cloud data warehouse services are preferred by many organizations as they are cheaper, easier to setup, and can offer superior performance compared to on premise systems.
Examples of major on premise data warehouses
- Teradata
- Oracle Exadata
- SAP Business Warehouse
Examples of major cloud data warehouses
- Amazon Redshift – enables possibly the fastest access to petabyte-scale data
- Google BigQuery – fully managed infrastructure with no setup and a fast learning curve
- Panoply – a cloud data warehouse that automatically transforms and optimizes data
Data warehouse use cases
Some examples of how enterprises use data warehouses include:
- Customer/patient analysis
- Operational reporting
- Strategic decision-making
Big Data and Data Analytics in Healthcare
Big data is a term that describes extremely large volumes of data gathered over short or long periods of time. Healthcare is one of the main areas in which big data is collected. Traditional database systems have a hard time processing and analyzing such large data stores, which has led to the emergence of new tools and infrastructures.
Big Data Analytics in Healthcare
- Medical image processing—CT scans, MRI scans, X-rays, and ultrasounds are imaging techniques we've all heard of for diagnosis and treatment planning. Systems need to be in place that can process the flood of data produced by different medical imaging techniques.
- Genomics—Analyzing genome-scale data is an important challenge in computational biology that could lead to improved care for patients with personalized treatments.
- Clinical operations—Research to determine more clinically relevant and cost-effective ways to diagnose and treat patients requires big data analyses.
- Research & development—Statistical tools can help improve clinical trial design and patient recruitment, better matching treatments to individual patients by analyzing huge stores of data from historical clinical trials and patient records.
- Public health—Big data analysis can identify disease patterns and disease outbreaks to improve public health surveillance, develop targeted vaccines quicker, and prevent public health crises.
What Do Telehealth and Data Warehousing Have in Common?
In Telehealth as in all of medicine, there is a need for descriptive, predictive, and prescriptive analyses on large stores of patient data to make informed data-driven medical decisions.
A data warehouse provides a platform to integrate data warehousing and data mining techniques in the telehealth area, from which a decision-support platform can be built. These decision support tools can, in turn, be used to improve diagnostic and treatment decisions and improve patient care.
There are various sources of data useful for building a Telehealth decision-support system, including, but not limited to, patient-specific data, pharmaceutical data, length of hospital stays, and treatment costs.
Evidence-based medicine relies on published clinical evidence. A clinical data warehouse can provide the necessary cross-institutional data required to design and conduct clinical trials, develop care protocols, generate practice guidelines and standards, and carry out other types of analyses such as those involved in cost-benefit analyses that require access to the wide variety of data collected for every patient encounter with a healthcare system.
Examples of Data Warehousing in Telehealth
- Choosing Treatments: Medical image data, for example, can range anywhere from a few megabytes to hundreds of megabytes per scan. Such data require a system with a large capacity for long-term storage and high-performance computing power for rapid analysis. Applications such as telestroke require rapid access to these complex imaging data sets, and with advances in image-based analytics to assess the type and extent of the stroke, rural sites can better triage which hospitals to send a patient to for optimal care.
- Patient Home Monitoring: The transmission of patient vital signs and other data through IoT (Internet of Things) devices or special software can assist with patient monitoring. All this data needs somewhere to go, and a data warehouse is optimal. Data analytics tools can be implemented to monitor these data and alert healthcare providers when important clinical indicators are beyond baseline or normal variation, requiring an intervention.
- Genomics: The P4 Medicine initiative uses a systematic approach to analyze genome-scale datasets to determine disease states, explore new approaches to drug treatments, and proactively treat people with telehealth methods. Using such high-density data for exploration, discovery, and clinical decisions demands robust data processing and analysis systems, such as data warehouses.
Managing Big Data in Telehealth: Security Issues
The adoption of big data and data warehousing in telehealth significantly increases security and patient privacy concerns. Patient information is stored in data centers with varying levels of security. Most healthcare data centers have HIPAA certification, but that certification does not guarantee patient record safety.
Furthermore, traditional database security solutions cannot be directly applied to large and diverse data stores. Appropriate healthcare governance is necessary before using data for analytics.
Real-time security analytics
The healthcare industry is witnessing a deluge of sophisticated attacks ranging from Distributed Denial of Service (DDoS) to stealthy malware, such as in the case of the 2017 WannaCry attack, which put significant strain on the U.K.'s public healthcare provider, the NHS. The potential for such attacks must be taken into account when designing any security solution—real-time security analytics can help prevent and deal with such attacks.
Preserving privacy
Data anonymization prior to analytics could protect patient identity. As the telehealth industry leverages IoT devices to transmit vital signs to healthcare cloud-based systems, there is a need to process and analyze this data while preserving patient privacy.
Additionally, as healthcare analytics gains popularity, new privacy laws need to be drafted to ensure patient privacy. New laws need to be drafted to clearly illustrate all processes involved in performing big data analytics on patient data.
Closing Thoughts
- As the volumes of data collected by organizations rapidly increase, there is a need to extract important information from that data. For telehealth, we need strong evidence-based decisions based on many data sources when choosing optimum patient treatments.
- Data warehousing and big data analytics are exciting technologies that address the problem of processing, storing, and analyzing the vast data stores and sources needed for intelligent telehealth decision support.
Image Attribution:
[1]Translational Research Institute
[2]Centogene